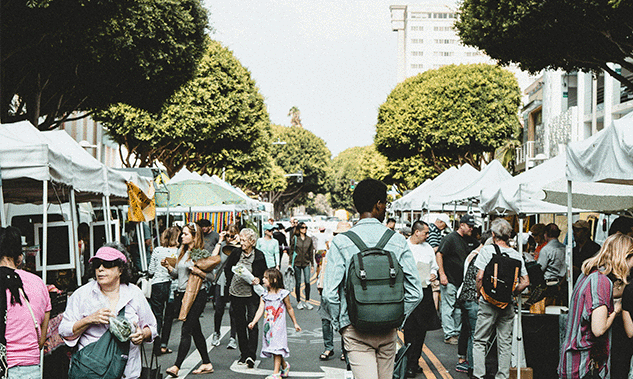
DISCOVER: HELPING INFORM URBAN DESIGN WITH DATA
Designing the move from a prop tech enabled consultancy into a product led growth model.
​
My team and I were tasked with taking a data-rich urban life report and the accompanying data investigation tool and turning it into a user experience that enriched a much wider audience and a robust sales tool.
Role: Product Designer/Product Owner/Product Manager
​
Credits: Founder & CEO & SME: Jess Christiansen-Franks & Lucinda Hartly / CTO: Gregor Paknic / Data & Analytics Lead: Josh Trew / Full Stack Dev: Amy Hawkes / Senior Front End Engineer: Di MacDonald / Junior Full Stack Dev: Will Hodge / Data Scientist: Dhanya Maheswaran
0 to 1: Creating and launching Discover
Neighbourlytics
Neighbourlyics is an urban analytics company that aims to solve the human data gap for place-makers.
The product has been created for Planners, Designers and Asset Managers within city councils, real estate developers and private investors as well as consultants that are often brought on as part of a project team.

UI Concepts based on current product
Context
The goal/problem space
​
Neighbourlytics was a tech-enabled consultancy looking to develop a sales-led product offer (with minimal consultancy work) in order to achieve growth as a product-led company.
To do this, we needed to make a product-led growth model that would ultimately lead users to purchase based on their own experienced value and structured onboarding.

Customers had to buy first to see data
Hypothesis: Customers would purchase reports faster if they were able to see some relevant data first

Existing users expected all the insights to be found for them

Product was created to be shown through by a human

Time to sale was slow! On average about 8 months
Hypothesis: If we went to a PLG growth model, we’d need to expand our ICP reach away from just Evangelists and Early Adopters
Hypothesis: If customers could onboard themselves, the sales team would be freed up for more sales work
Goal: To bring down the average time to sale
The challenges
Our customers
​
Internally, there were years of built-in assumptions around who the users actually were and how/why they needed to use the product.
​
​
​
The debt of complexity
​
The platform also had many years of UX debt and a complex offering suited to the consultancy model plus a long sales process which meant all of the existing user-base had had their hands held throughout the entire onboarding process and nothing was made for instant access.
Misalignment with current users to future ones
​
-
Before the conversion to PLG started, work had been done to identify a small set of ideal customer profiles and was being used extensively to make decisions around the service design, product design and go-to-market strategy.
​
-
This led to many unvalidated ideas being shipped and low stickiness levels even for the then current users.

What can we automate in the product so we can grow exponentially?
An exclusive, static and costly product
​
-
Users encountered many hurdles in the product like complex metrics presented statically and an overuse of industry technical jargon.
​
-
The interactive tool’s interface was unintuitive and required 1:1 training but the biggest barrier was by far the cost to both users and the business.
​
-
Reports were manually created by the data team and customers needed a lot of support just to see and use their ordered data.

If we go to PLG who will our new market be?
My approach: Discovery & definition
Planning/Product
Collaborating with the CEO + CTO on direction, then planning what the immediate future looked like

Research/Discovery
Discovery interviews 

Desktop studies

Innovation session

Flows + wireframing
Taking cues and ideas from the interviews, research studies and innovation session, creating low and mid-fi wireframe for skeleton
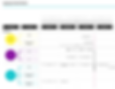

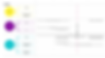
Planning
​
I organised a couple of strategic planning sessions with the leadership to define a goals timeline, MVP roadmap and a basic features Kanban board was also setup.
With my Product Manager hat on, I worked closely with our CTO to understand tech timelines, requirements and limitations.
I also spent time with the data team and SMEs (Founders) defining what data was currently on offer vs what we could offer to our future users.
​
Research Plan
​
I set out on a discovery mission to understand what was needed to move into a PLG model. I was able to recruit the help of UX researchers and with the input of our Sales & Marketing team lead I set about understanding:
the property tech market
Desktop studies
competitors and analogous product research
Innovation session
our total addressable market and redefining who our ICPs were in the new PLG model
Innovation session
barriers to current sales
Discovery interviews
how our target users consumed data and tech in the current market
Discovery interviews
Research method: Discovery interviews
​
To gain deep insight, we conducted 12 x 45 minute moderated discovery sessions. These were the things we wanted to find out:
​
-
Find out what metrics users are looking for in property development projects
-
Test brand value assumption that users see value in community intelligence data to inform decision-making
-
Test product value assumption that users have a need for community intelligence (CI) data everywhere to explore various geographies and inform decision-making
-
Prioritise feasible analytics metrics and most desirable CI metrics


Key finding: Users were comfortable navigating data from the ABS and most had used it before.
Our lightbulb moment was to make sure ABS data was the first metric seen when users landed in the new platform so it could serve as both a guiding light on how to use the overlays, and an orienting feature.
Research method: Innovation session
​
To help kick off my research, get team buy-in and encourage involvement, I created an async innovation session with them to get the juices flowing. The main things I got the team’s input into were:
-
Thoughts on secondary and tertiary markets (started thinking about TAM)
-
What could the layout look like to a user? Dashboard? Interactive map?
-
Who were our competitors plus anything cool that would fit.


Key finding: The hive mind effect was invaluable in kicking off my research as most of the team had been at the company much longer and had a lot of context around things like our total addressable market, competitors and data visualisations.
Flows and wireframes
​
Taking all the collected discovery insights, I then worked towards basic flows and low fi wireframes. These were needed to inform engineering on what to start building.

Experience ideas from ideation session (low-fidelity)
2 concepts shown



Mid-fidelity wireframes - technical limitations testing
9 wireframes shown




My approach: Development & delivery
From wireframes to prototyping
​
After engineering had released the 'walking skeleton' based on the mid-fidelity wireframes, it was then a matter of adapting the styles that were concurrently being created for the other major product in the suite; the reports.
​
​
Initiating the conversation with customers
​
I collaborated with our marketing/sales lead to help them understand what the new product was offering and how it worked in conjunction with the existing products.
High fidelity prototype - styled designs for dev grooming
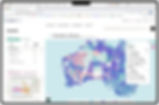
User lands and can pan/hover around. Hover data is shown in popover window over map.
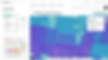
User zooms, selects a different overlay, minimises popover accordions
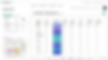
User selects ‘Suburb side-by-side view’ button
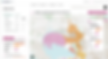
User selects Visitation panel
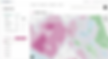
User selects Movement panel

Popover accordion and minimise data shown
Closed launch and ‘friendlies’ invites
​
We released as a closed beta and asked our existing (and friendly) customer base to take a look and provide feedback on the new product - about 40 users were approached.



Screenshots of the closed release product
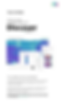
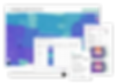
Email example to ‘friendlies’
Prototype display pack for marketing/sales use

Landing page/intro to product suite ideas - product architecture
My approach: Iterative development + measurement
Testing and iterations
Test with fresh-eyed users, collate feedback from ‘friendlies’, iterate with view to launch 1.1

Launch + measurement setup
Launch via email/link invite, setup GA4, Hubspot and Hotjar points for measurement

Platform integration + onboarding
Collaborate on best way for Discover to integrate into platform as a whole and take steps to help users onboard after receiving link to enter.
Research method: Contextual interviews (Customer + usability)
​
I conducted 10 contextual interviews outside of our customer base with industry ICPs. During this testing phase I spent time collecting data around user journeys, JTBD and then took participants through the new product.
User journeys were created and a directional set of iterations were made based on the comments we were getting back from our closed release to current customers alongside the interviews.
Research objectives: (What do we want to know or better understand?)
-
Where and how in the customer journey Discover is being/could be used (to be synthesised)
-
Which ABS stats are the most important (to least important) at each stage of the journey mentioned.
-
What aspects in Discover could be improved
-
What would an expected price point be?
Interviews analysis and pattern finding
5 pattern groups shown





Key finding: Users assumed our behavioural data sets were from the ABS due to a lack of signalling and information in the UI, but were really interested in the data when they found out it was from very recent mobile sources.
Iterations
​
From the contextual interviews, I was able to narrow down the next set of iterations and UI changes to go into Dev.

Please note - this image is for illustrative purposes only! This is not how I gave the changes to Dev.
High-fidelity iterated prototypes

User lands and can pan/hover around. Hover data is shown in popover window over map.

Screen shows when user has selected a suburb then taps Visitors tab on behavioural data panel.